Path overview
In this path, you’ll learn the fundamentals of R and build upon them with more advanced skills. You’ll learn how to use RStudio, applications and tools, tidyverse, DataFrames, tibbles, operators, expressions, and much more — as well as data visualization, graphs, plots, and charts.
Best of all, you’ll learn by doing — you’ll write code and get feedback directly in the browser. You’ll apply your skills to several guided projects involving realistic business scenarios to build your portfolio and prepare for your next interview.
Key skills
- Programming with R to perform complex statistical analysis of large datasets
- Performing SQL queries and web-scraping to explore and extract data from databases and websites
- Performing efficient data analysis from start to finish
- Building insightful data visualizations to tell stories
Path outline
Part 1: Introduction to R [4 courses]
Introduction to Data Analysis in R 3h
Objectives- Define R programming syntax
- Define variable use and naming rules
- Perform calculations using arithmetic operators
Data Structures in R 6h
Objectives- Create a data structure
- Index a data structure
- Perform operations over a data structure
Control Flow, Iteration, and Functions in R 4h
Objectives- Employ control flow with if-else statements
- Replicate your code using iteration
- Write functions
Specialized Data Processing in R 4h
Objectives- Manipulate strings from the stringr package
- Manipulate strings from the lubridate package
- Employ the map function from the purrr package
Part 2: Data Visualization in R [1 course]
Introduction to Data Visualization in R 4h
Objectives- Visualize changes over time using line graphs
- Analyze data distributions using histograms
- Compare groups using bar charts and box plots
- Identify the relationships between variables using scatter plots
Part 3: Data Cleaning in R [2 courses]
Introduction to Data Cleaning in R 7h
Objectives- Manipulate DataFrames
- Define relational data
- Resolve missing data
- Reshape data using the tidyr package
Advanced Data Cleaning in R 6h
Objectives- Employ regular expressions to clean and manipulate text data
- Employ the map and anonymous functions
- Resolve missing data
Part 4: Working with Data Sources Using SQL [6 courses]
Introduction to SQL and Databases 5h
Objectives- Define the structure of SQL
- Create basic queries to extract data from tables in a database
- Define databases
- Identify different versions of SQL
- Write good SQL code
Summarizing Data in SQL 3h
Objectives- Employ SQL to compute statistics
- Provide statistics by group
- Filter results over groups
Combining Tables in SQL Course 3h
Objectives- Combine tables using inner joins
- Employ different types of joins
- Employ other SQL clauses with joins
- Join on complex conditions
- Employ set operators like UNION and EXCEPT
Querying Databases with SQL and R 1h
Objectives- Connect to a SQLite database using R
- Query a SQLite database using R
- Retrieve a subset of data
SQL Subqueries 6h
Objectives- Nest a query inside another query
- Employ different types of subqueries
- Employ common table expressions
- Scale your project with complex queries
Window Functions in SQL 5h
Objectives- Set up a frame for window functions
- Compute running aggregations with aggregate window functions
- Explore rank window functions
- Apply distribution window functions
- Use offset window functions
Part 5: APIs and Web Scraping in R [2 courses]
Introduction to APIs in R 3h
Objectives- Query external data sources using an API
- Query using an API with authentication
Introduction to Web Scraping in R 3h
Objectives- Scrape data from the web
- Identify tools for complex web pages
Part 6: Probability and Statistics [5 courses]
Introduction to Statistics in R 5h
Objectives- Sample data using simple random sampling, stratified sampling, and cluster sampling
- Measure variables in statistics
- Build, visualize, and compare frequency distribution tables
Intermediate Statistics in R 3h
Objectives- Summarize a distribution using the mean, the weighted mean, the median, or the mode
- Measure the variability of a distribution using the variance and the standard deviation
- Compare values using z-scores
Introduction to Probability in R 1h
Objectives- Estimate theoretical and empirical probabilities
- Define the fundamental rules of probability
- Identify combinations and permutations
Conditional Probability in R 2h
Objectives- Assign probabilities based on conditions
- Assign probabilities based on event independence
- Assign probabilities based on prior knowledge
- Create spam filters using multinomial Naive Bayes
Hypothesis Testing in R 1h
Objectives- Implement probability density functions
- Create testable hypotheses
- Decide which hypotheses to support based on your data
Part 7: Predictive Modeling and Machine Learning in R [2 courses]
Linear Regression Modeling in R 3h
Objectives- Define predictive modeling
- Build linear regression models
- Interpret linear regression models
- Assess model fit and accuracy
Introduction to Machine Learning in R 2h
Objectives- Identify a proper machine learning workflow
- Implement the k-nearest neighbors algorithm
- Employ the caret library
Part 8: Shiny Applications in R [1 course]
Introduction to Interactive Web Applications in Shiny 2h
Objectives- Read the structure of a Shiny app
- Program inputs and outputs in a Shiny interface
- Extend your Shiny apps
The Dataquest guarantee
Dataquest has helped thousands of people start new careers in data. If you put in the work and follow our path, you’ll master data skills and grow your career.
We believe so strongly in our paths that we offer a full satisfaction guarantee. If you complete a career path on Dataquest and aren’t satisfied with your outcome, we’ll give you a refund.
Master skills faster with Dataquest
Go from zero to job-ready
Learn exactly what you need to achieve your goal. Don’t waste time on unrelated lessons.
Build your project portfolio
Build confidence with our in-depth projects, and show off your data skills.
Challenge yourself with exercises
Work with real data from day one with interactive lessons and hands-on exercises.
Showcase your path certification
Share the evidence of your hard work with your network and potential employers.
Projects in this path
Project: Install RStudio
For this project, we’ll step into the role of aspiring data scientists, setting up our programming environment by installing R and RStudio. We’ll explore RStudio’s key features for efficient R programming and data analysis.
Guided Project: Investigating COVID-19 Virus Trends
For this project, you’ll be a data analyst investigating a real COVID-19 dataset. You’ll use R to manipulate and analyze the data, building in-demand skills as you find countries with the highest positive test rates.
Guided Project: Creating An Efficient Data Analysis Workflow
For this project, you’ll act as a data analyst for a company selling programming books to analyze sales data and extract useful insights to determine the most profitable titles.
Guided Project: Creating An Efficient Data Analysis Workflow, Part 2
For this project, you’ll be a data analyst at a book company using R to clean sales data and analyze if a new program successfully boosted purchases and review sentiment.
Guided Project: Analyzing Forest Fire Data
For this project, we’ll step into the role of data analysts to explore a dataset on forest fires. Using R and data visualization techniques, we’ll analyze trends and factors related to fire occurrence and severity.
Plus 14 more projects
Learning resources
Grow your career with
Dataquest.
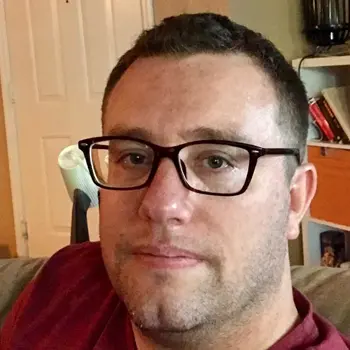
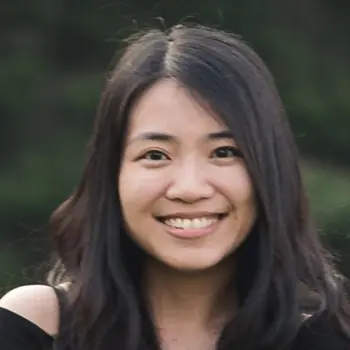
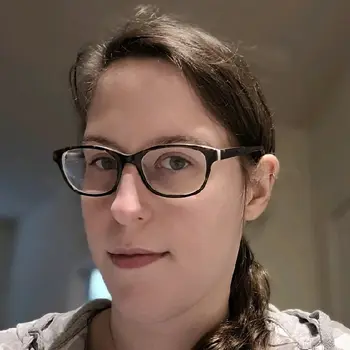