How to Become a Data Scientist: A Personal Journey
If you want to know how to become a data scientist, then you’re in the right place. I’ve been where you are, and now I want to help. Starting a career in data science from scratch can seem daunting, but it's definitely achievable. A decade ago, I was just a college graduate with a history degree. I then became a machine learning engineer, data science consultant, and the founder of Dataquest.
If I could do everything over, I would follow the steps I’m going to share with you in this article. It would have fast-tracked my career, saved me thousands of hours, and prevented a few gray hairs.
Your Questions, My Answers
But you might be wondering: Is it still worth pursuing a career in data science? Will AI replace data scientists, or will the role evolve alongside it? What are the essential AI skills you need to thrive in this field? Let me answer these questions and set you on the right path.
Is data science still a good career choice?
Yes — a data science career is still a fantastic choice. Demand for data scientists is high, and the world is generating a massive (and increasing) amount of data every day.
We don't claim to have a crystal ball or know what the future holds, but data science is a fast-growing field with high demand and lucrative salaries.
Will AI replace data scientists?
AI is unlikely to replace data scientists entirely. But the role of a data scientist will evolve significantly with the integration of AI. Data scientists will increasingly rely on AI-driven insights for faster and more accurate data-driven decision-making, focusing on strategic analysis. They will collaborate closely with AI engineers and machine learning specialists in developing and fine-tuning AI models, encompassing algorithm selection, feature engineering, and ethical considerations. To effectively work with AI specialists, data scientists will expand their skill set to include interdisciplinary knowledge, including machine learning, deep learning, and natural language processing. Additionally, data scientists will play a pivotal role in ensuring ethical AI use, addressing bias, data privacy, and ethical principles. Continuous learning will be vital to stay current in this rapidly evolving field.
What are the AI skills a data scientist needs?
Every data scientist needs to know the basics, but now that it's everywhere, data scientists also need to acquire essential AI-related skills. This includes a strong grasp of machine learning concepts, deep learning frameworks like TensorFlow and PyTorch, proficiency in natural language processing (NLP) for text analysis, and a deep understanding of AI ethics and bias mitigation. Data scientists should also be familar with AI development tools and libraries, possess data engineering skills, and thrive in interdisciplinary, collaborative environments. Continuous learning to stay on top of AI advancements is assumed. While AI won't replace data scientists, these skills will enable them to contribute effectively to AI-driven projects.
The Wrong and Right Way
When I was learning, I tried to follow various online data science guides, but I ended up bored and without any actual data science skills to show for my time.
The guides were like a teacher at school handing me a bunch of books and telling me to read them all — a learning approach that never appealed to me. It was frustrating and self-defeating.
Over time, I realized that I learn most effectively when I'm working on a problem I'm interested in.
And then it clicked.
Instead of learning a checklist of data science skills, I decided to focus on building projects around real data. Not only did this learning method motivate me, it also mirrored the work I’d do in an actual data scientist role.
I created this guide to help aspiring data scientists who are in the same position I was in. In fact, that’s also why I created Dataquest. Our data scientist career path is designed to take you from beginner to job-ready in less than a year using actual code and real-world projects.
However, a series of courses isn’t enough. You need to know how to think, study, plan, and execute effectively if you want to become a data scientist. This actionable guide contains everything you need to know.
How to Become a Data Scientist:
- Step 1: Question Everything
- Step 2: Learn The Basics
- Step 3: Build Projects
- Step 4: Share Your Work
- Step 5: Learn From Others
- Step 6: Push Your Boundaries
Now, let’s go over each of these one by one.
Step 1: Question Everything
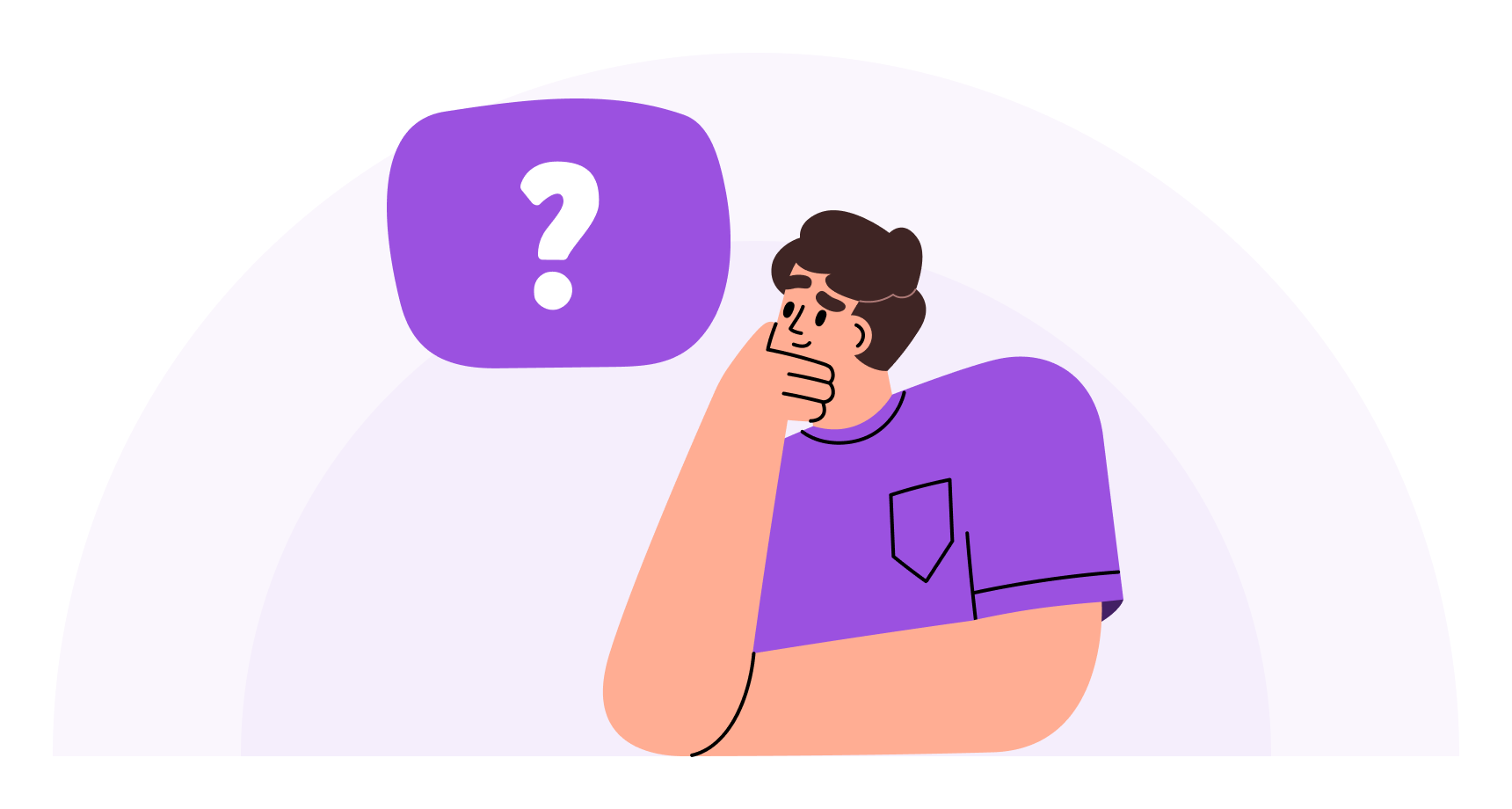
The data science and data analytics field is appealing because you get to answer interesting questions using actual data and code. These questions can range from Can I predict whether a flight will be on time? to How much does the U.S. spend per student on education?
To answer these questions, you need to develop an analytical mindset.
The best way to develop this mindset is to start with analyzing news articles. First, find a news article that discusses data. Here are two great examples: Can Running Make You Smarter? or Is Sugar Really Bad for You?
Then, think about the following:
- How they reach their conclusions given the data they discuss
- How you might design a study to investigate further
- What questions you might want to ask if you had access to the underlying data
Some articles, like this one on gun deaths in the U.S. and this one on online communities supporting Donald Trump actually have the underlying data available for download. This allows you to explore even deeper. You could do the following:
- Download the data, and open it in Excel or an equivalent tool
- See what patterns you can find in the data by eyeballing it
- Do you think the data supports the conclusions of the article? Why or why not?
- What additional questions do you think you can use the data to answer?
Here are some good places to find data-driven articles:
Reflect
After a few weeks of reading articles, reflect on whether you enjoyed coming up with questions and answering them. Becoming a data scientist is a long road, and you need to be very passionate about the field to make it all the way.
Data scientists constantly come up with questions and answer them using mathematical models and data analysis tools, so this step is great for understanding whether you'll actually like the work.
When in Doubt, Analyze Things You Enjoy
Perhaps you don't enjoy the process of coming up with questions in the abstract, but maybe you enjoy analyzing health or finance data. Find what you're passionate about, and then start viewing that passion with an analytical mindset.
Personally, I was very interested in stock market data, which motivated me to build a model to predict the market.
If you want to put in the months of hard work necessary to learn data science, working on something you’re passionate about will help you stay motivated when you face setbacks.
Step 2: Learn The Basics
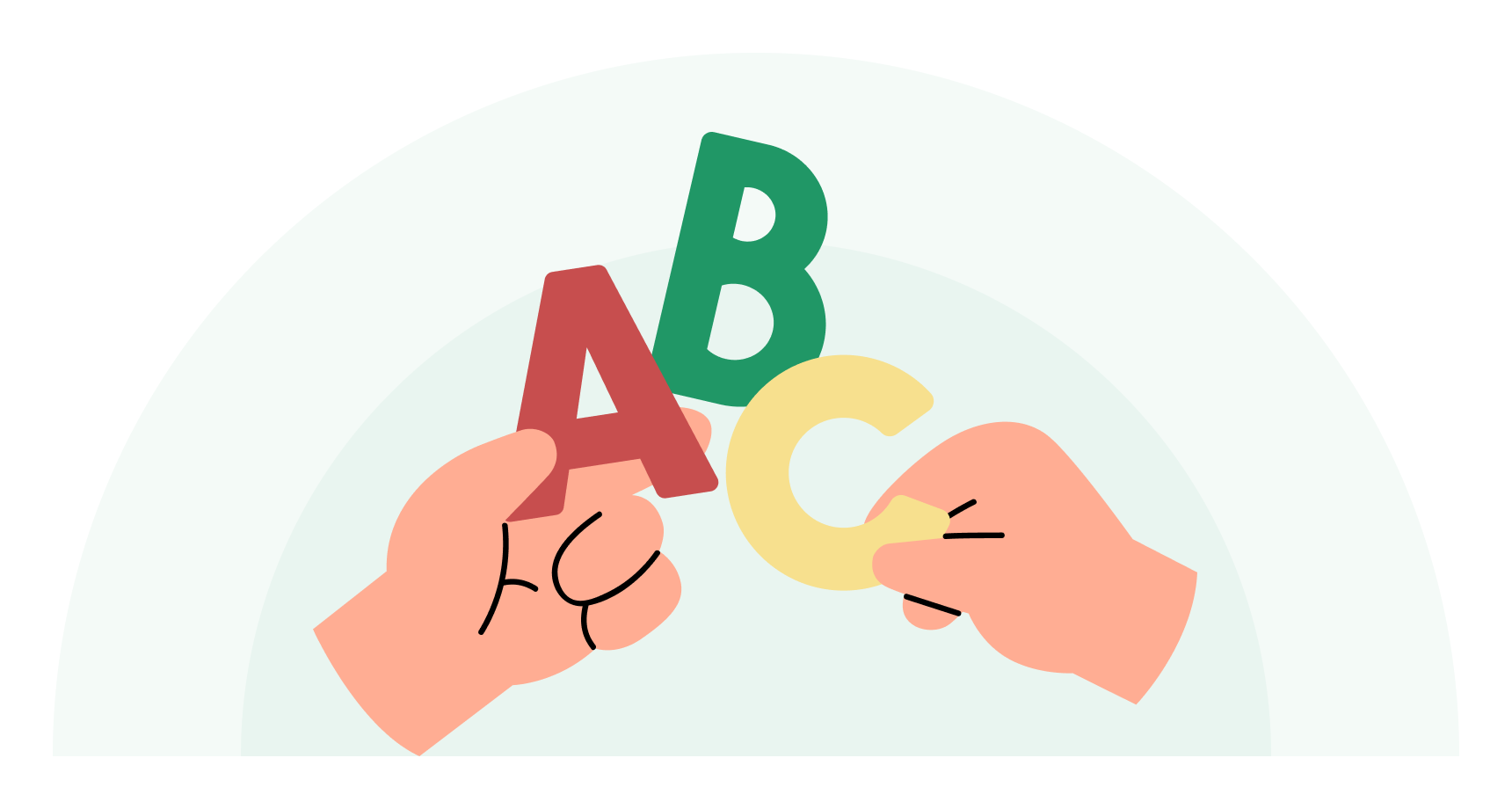
Once you've figured out how to ask the right questions, you're ready to start learning the technical skills necessary to answer them. I recommend learning data science by studying the basics of programming in Python.
Python is a programming language that has consistent syntax and is often recommended for beginners. It’s also versatile enough for extremely complex data science and machine learning-related work, such as deep learning or artificial intelligence using big data.
Many people worry about which programming language to choose, but here are the key points to remember:
- Data science is about answering questions and driving business value, not about tools
- Learning the concepts is more important than learning the syntax
- Building projects and sharing them is what you'll do in an actual data science role, and learning this way will give you a head start
Super important note: The goal isn’t to learn everything; it’s to learn just enough to start building projects.
Where You Should Learn
Here are a few great places to learn:
- Dataquest — I started Dataquest to make learning Python for data science or data analysis easier, faster, and more fun. We offer basic Python fundamentals courses, all the way to an all-in-one path consisting of all courses you need to become a data scientist.
- Learn Python the Hard Way — a book that teaches Python concepts from the basics to more in-depth programs.
- Python.org — a free tutorial provided by the main Python site.
The key is to learn the basics and start answering some of the questions you came up with over the past few weeks browsing articles.
Step 3: Build Projects
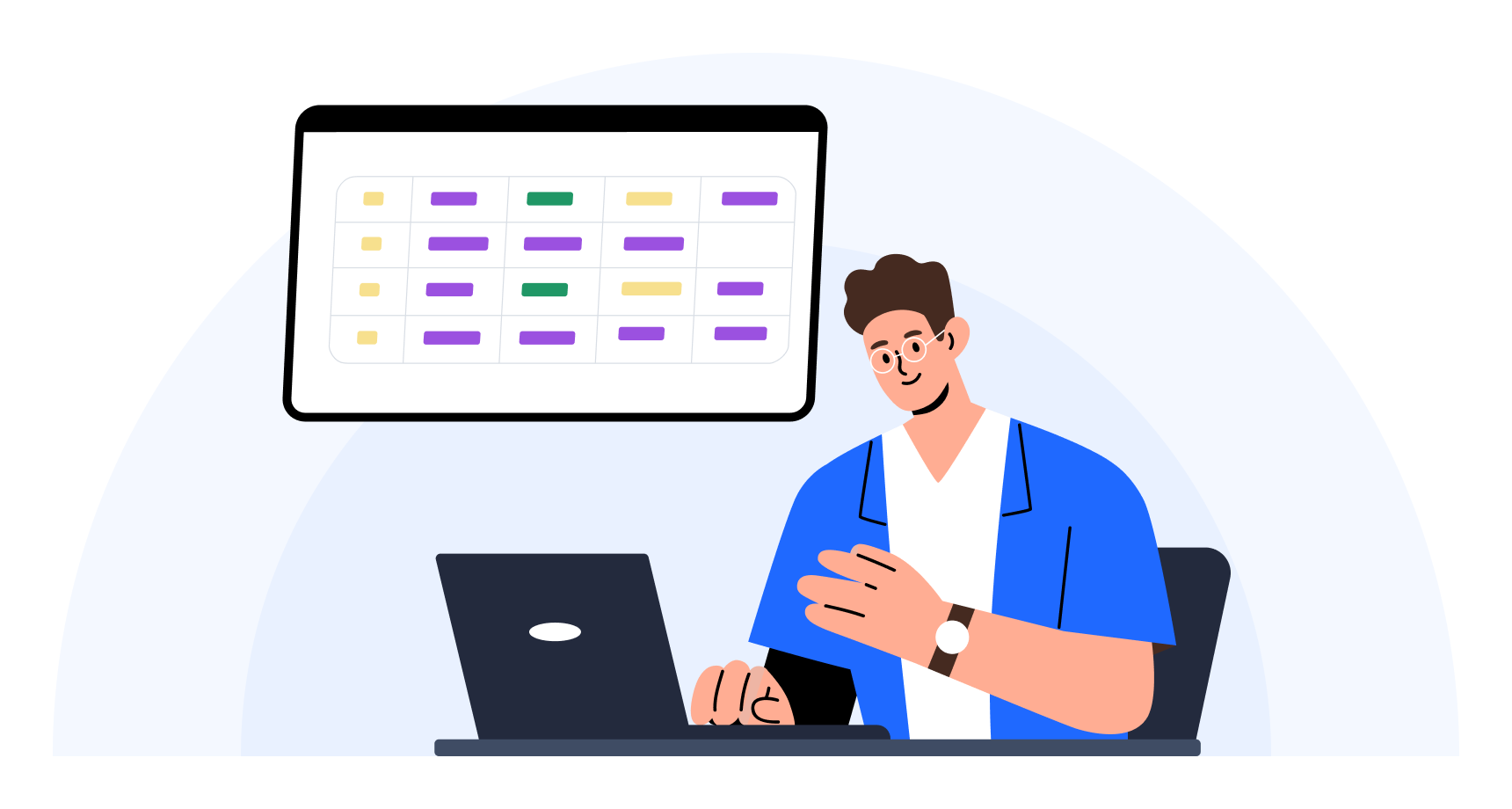
As you're learning the basics of coding, you should start building projects that answer interesting questions that will showcase your data science skills.
The projects you build don't have to be complex. For example, you could analyze Super Bowl winners to find patterns.
The key is to find interesting datasets, ask questions about the data, then answer those questions with code. If you need help finding free datasets for your projects, we've got you covered!
As you're building projects, remember that:
- Most data science work is data cleaning.
- The most common machine learning technique is linear regression.
- Everyone starts somewhere. Even if you feel like what you're doing isn't impressive, it's still worth working on.
Where to Find Project Ideas
Not only does building projects help you practice your skills and understand real data science work, it also helps you build a portfolio to show potential employers.
Here are some more detailed guides on building projects on your own:
Additionally, most of Dataquest’s courses contain interactive projects that you can complete while you’re learning. Here are just a few examples:
- Profitable App Profiles for the App Store and Google Play Markets — Explore the app market by analyzing what makes an app profitable across both iOS and Android platforms, focusing on the potential of book-based apps enhanced with unique features.
- Exploring Hacker News Posts — Work with a dataset of submissions to Hacker News, a popular technology site.
- Exploring eBay Car Sales Data — Use Python to work with a scraped dataset of used cars from eBay Kleinanzeigen, a classifieds section of the German eBay website.
- Star Wars Survey — Work with Jupyter Notebook to analyze data on the Star Wars movies.
- Analyzing NYC High School Data — Discover the SAT performance of different demographics using scatter plots and maps.
- Classifying Heart Disease — Go through the complete machine learning workflow of data exploration, data splitting, model creation, and model evaluation to develop a logistic regression classifier for detecting heart disease.
Add Project Complexity
After building a few small projects, it's time to kick it up a notch! We need to add layers of project complexity to learn more advanced topics. At this step, however, it's crucial to execute this in an area you're interested in.
My interest was the stock market, so all my advanced projects had to do with predictive modeling. As your skills grow, you can make the problem more complex by adding nuances like minute-by-minute prices and more accurate predictions. Check out our article on Python projects ideas for more inspiration.
Step 4: Share Your Work
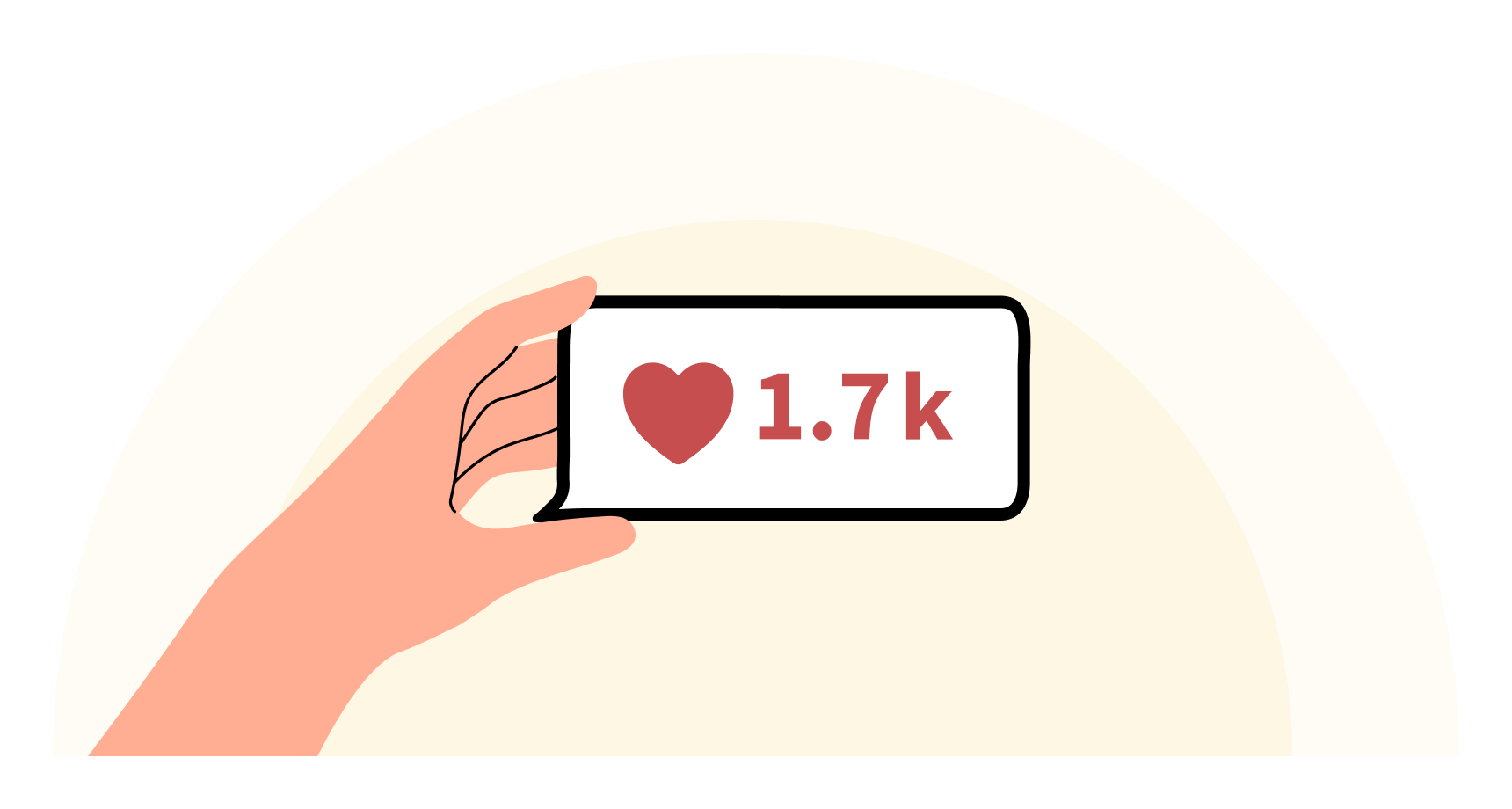
Once you've built a few data science projects, share them with others on GitHub!
Here’s why:
- It makes you think about how to best present your projects, which is what you'd do in a data science role.
- They allow your peers to view your projects and provide feedback.
- They allow employers to view your projects.
Helpful resources about project portfolios:
- How To Present Your Data Science Portfolio on GitHub
- Data Science Portfolios That Will Get You the Job
Start a Simple Blog
Along with uploading your work to GitHub, you should also think about publishing a blog. When I was learning data science, writing blog posts helped me do the following:
- Capture interest from recruiters
- Learn concepts more thoroughly (the process of teaching really helps you learn)
- Connect with peers
Here are some good topics for blog posts:
- Explaining data science and programming concepts
- Discussing your projects and walking through your findings
- Discussing how you’re learning data science
Here’s an example of a visualization I made on my blog many years ago that tries to answer the question: do the Simpsons characters like each other?
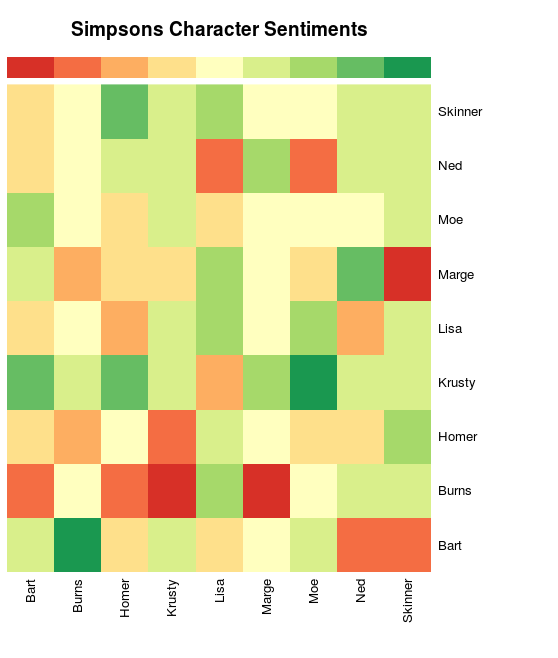
Step 5: Learn From Others
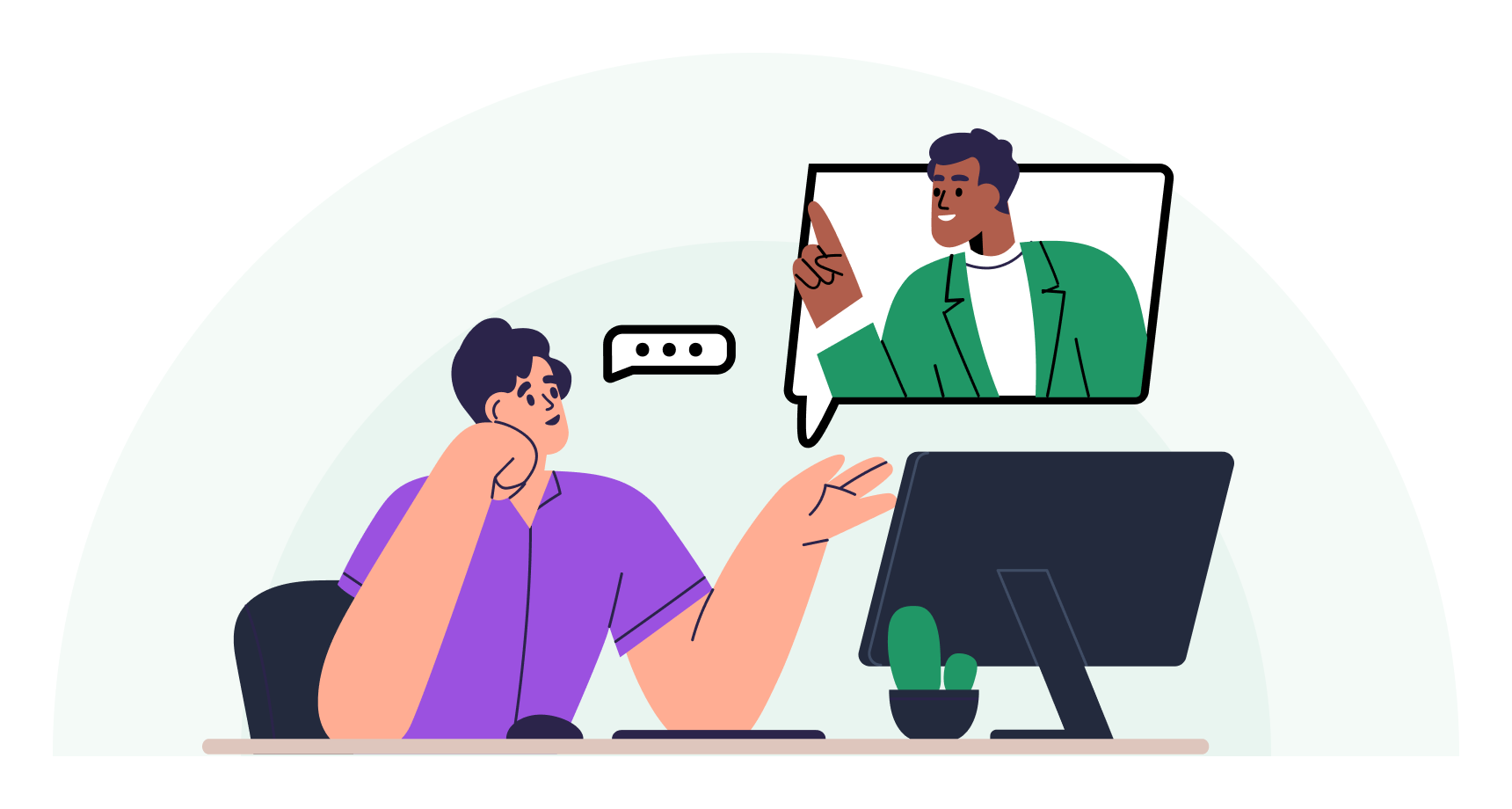
After you've started to build an online presence, it's a good idea to start engaging with other data scientists. You can do this in-person or in online communities. Here are some good online communities:
Here at Dataquest, we have an online community that learners can use to receive feedback on projects, discuss tough data-related problems, and build relationships with data professionals.
Personally, I was very active on Quora and Kaggle when I was learning, which helped me immensely. Engaging in online communities is a good way to do the following:
- Find other people to learn with
- Enhance your profile and find opportunities
- Strengthen your knowledge by learning from others
You can also engage with people in-person through Meetups. In-person engagement can help you meet and learn from more experienced data scientists in your area.
Step 6: Push Your Boundaries
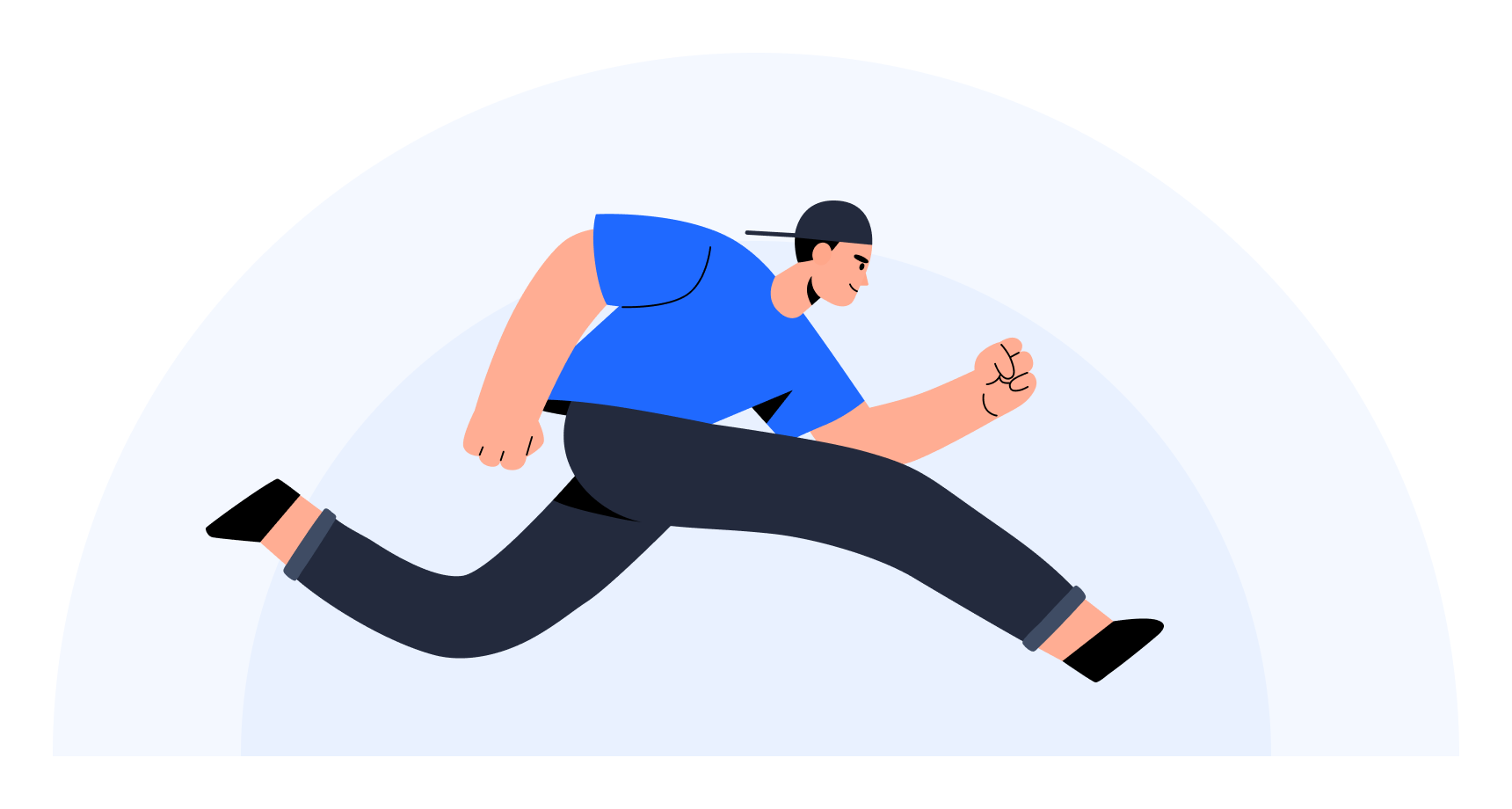
What kind of data scientists to companies want to hire? The ones that find critical insights that save them money or make their customers happier. You have to apply the same process to learning — keep searching for new questions to answer, and keep answering harder and more complex questions.
If you look back on your projects from a month or two ago, and you don’t see room for improvement, you probably aren't pushing your boundaries enough. You should be making strong progress every month, and your work should reflect that.
Here are some ways to push your boundaries and learn data science faster:
- Try working with a larger dataset
- Start a data science project that requires knowledge you don't have
- Try making your project run faster
- Teach what you did in a project to someone else
You’ve Got This!
Studying to become a data scientist or data engineer isn't easy, but the key is to stay motivated and enjoy what you're doing. If you're consistently building projects and sharing them, you'll build your expertise and get the data scientist job that you want.
I haven't given you an exact roadmap to learning data science, but if you follow this process, you'll get farther than you imagined you could. Anyone can become a data scientist if you're motivated enough.
After years of being frustrated with how conventional sites taught data science, I created Dataquest, a better way to learn data science online. Dataquest solves the problems of MOOCs, where you never know what course to take next, and you're never motivated by what you're learning.
Dataquest leverages the lessons I've learned from helping thousands of people learn data science, and it focuses on making the learning experience engaging. At Dataquest, you'll build dozens of projects, and you’ll learn all the skills you need to be a successful data scientist. Dataquest students have been hired at companies like Accenture and SpaceX .
I wish you all the best on your path to becoming a data scientist!