How to Learn Python (Step-by-Step)
It was hard for me to learn Python, but it didn't have to be.
A decade ago, I was a fresh college grad armed with a history degree and not much else. Fast forward to today, and I'm a successful machine learning engineer, data science and deep learning consultant, and founder of Dataquest.
These days, I'm working on deep learning projects that keep me curious and motivated, constantly challenging me to learn and grow while creating tools that others can benefit from. But let me be real with you—it wasn't all smooth sailing to get to where I am now. My Python learning journey was long, filled with setbacks, and often frustrating.
If I’d known back then what I know now, I could have fast-tracked my career, saved thousands of hours, and avoided a whole lot of stress. Looking back, I’d take a completely different approach. That’s exactly why I wrote this article—to share the steps you need to learn Python the right way.
Based on my experience, this is the only guide you'll need on how to learn Python from scratch. Read it, bookmark it, and then read it again.
Why Most New Learners Fail to Learn Python
Many people struggle with learning Python, not because it’s inherently hard, but because they’re using the wrong approach or resources. The good news is that with the right approach, learning Python can be easier—and even enjoyable.
The Problem With Most Learning Resources
Many of the courses out there make learning more difficult than it has to be. Let me give you a personal example to illustrate my point.
When I first started learning to program, I wanted to do the things that excited me, like making websites or working with AI. Unfortunately, the course I was taking forced me to spend months on boring syntax. It was agony!
Throughout the course, Python code continued to look foreign and confusing. It was like an alien language. It’s no surprise I quickly lost interest.
Unfortunately, most Python learning resources are built this way. They assume you need to learn all of Python syntax before you can start doing anything interesting. This is why most new learners give up.
An Easier Way
After many failed attempts, I found a process that works much better. Since I believe it's the best way to learn Python programming, it’s the approach I wish I had when I was starting out.
Here’s the short version: Don’t waste time trying to memorize every bit of Python syntax. Instead, focus on picking up the basics, then jump straight into a project that excites you. That’s where the real learning happens.
This approach minimizes the time spent on mundane tasks and maximizes the fun parts of learning. Think about analyzing some personal data, building a website, or creating an autonomous drone with artificial intelligence!
This better way of learning is how I built Dataquest. Our courses are designed to get you building projects as soon as possible with minimal time spent on the boring syntax stuff.
How Long Does It Take to Learn Python?
If you’re looking for a general answer, here it is: Learning Python basics may only take a few weeks. However, if you’re pursuing a career as a programmer or data scientist, you can expect it to take 4 to 12 months to learn enough advanced Python to be job-ready. (This estimate comes from learners who have taken our Python for Data Science career path.)
The personalized answer is that it depends on several factors like your prior experience and how much time you can dedicate to learning. The good news: it's probably less time than you think, if you take the right approach.
So, what is the best way to learn Python? The following five steps will show you how to focus on what matters, skip the boring stuff, and actually enjoy the process. Your journey to learning Python the right way starts now!
Step 1: Identify What Motivates You
With the right motivation, anyone can become highly proficient in Python programming.
As a beginner, I struggled to keep myself awake when trying to memorize syntax. However, when I needed to apply Python fundamentals to build an interesting project, I happily stayed up all night to finish it.
What’s the lesson here? You need to find what motivates you and get excited about it! When getting started with Python, find one or two areas that interest you and stick with them.
Here are some areas where Python really shines—take a look and see which ones spark your interest. In Step 3: Structured Projects, I’ll share resources to help you get started in each:
- Data Science and Machine Learning
- Mobile Apps
- Websites
- Video Games
- Hardware / Sensors / Robots
- Data Processing and Analysis
- Automating Work Tasks
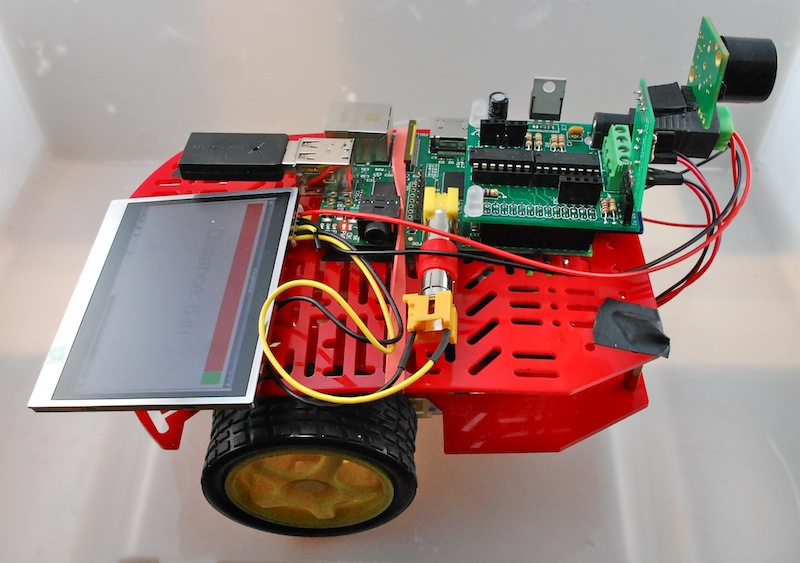
Step 2: Learn Python Basic Syntax, Quickly
I know, I know. I said we’d spend as little time as possible on syntax. Unfortunately, we can't avoid it entirely.
Here are some good resources to help you learn Python basic syntax without killing your motivation:
- Introduction to Python Programming Course — Our Python for beginners course that gets you coding quickly and encourages you to practice as you learn.
- Learn Python the Hard Way — A book that teaches Python concepts from the basics to more in-depth programs.
- Complete Guide to Python — Our comprehensive guide to Python consisting of tutorials, practice problems, a handy Python cheat sheet, guided projects, and frequently asked questions that will walk you through foundational Python concepts.
Again, I can’t emphasize this enough: Learn what syntax you can and move on. Ideally, you will spend a couple of weeks on this phase but no more than a month.
The truth is, most people pick up the syntax they need while working on projects that excite them—not by memorizing it upfront. Focus on getting the basics down quickly, and let your projects guide the rest. You'll be surprised how much you can learn just by doing.
Step 3: Start Doing Structured Projects
Once you’ve learned the basic Python syntax, start doing projects. Applying your knowledge right away will help you remember everything you’ve learned.
It’s better to begin with structured projects until you feel comfortable enough to create your own.
Guided Projects
Here are some examples of Dataquest structured (guided) projects. Which one ignites your curiosity?
- Building a Word-Guessing Game — Have some fun, and create a functional and interactive word-guessing game using Python.
- Building a Food Ordering App — Create a functional and interactive food ordering application using Python.
- Data Cleaning and Visualization, Star Wars-Style — Fans of Star Wars will not want to miss this structured project using real data from the movie.
- Web Scraping NBA Stats — Scrape, parse, and combine NBA statistics from the web.
- Predicting the Stock Market Using Machine Learning — Learn how to train a machine learning model for predicting the stock market.
- Predicting Heart Disease — Build a k-nearest neighbors classifier to predict whether patients might be at risk of heart disease.
- Detecting Pneumonia Using X-Ray Images — Build and train convolutional neural network (CNN) models to accurately classify whether an X-ray shows signs of pneumonia.
Structured Project Resources
Remember, there is no right place to start when it comes to structured projects. Let your motivations and goals guide you.
Are you interested in general data science or machine learning? Do you want to build something specific, like an app or website? Here are some recommended resources for inspiration, organized by category:
1. Data Science and Machine Learning
- Dataquest — Teaches you Python and data science interactively. You analyze a series of interesting datasets, ranging from CIA documents to NBA player stats to X-ray images. You eventually build complex algorithms, including neural networks, decision trees, and computer vision models.
- Scikit-learn Documentation — Scikit-learn is the main Python machine learning library. It has some great documentation and tutorials.
- CS109A — This is a Harvard class that teaches Python for data science. They have some of their projects and other materials online.
2. Mobile Apps
- Kivy Guide — Kivy is a tool that lets you make mobile apps with Python. They have a guide for getting started.
- BeeWare — Create native mobile and desktop applications in Python. The BeeWare project provides tools for building beautiful apps for any platform.
3. Websites
- Bottle Tutorial — Bottle is another web framework for Python. Here’s a guide for getting started with it.
- How To Tango With Django — A guide to using Django, a complex Python web framework.
4. Video Games
- Pygame Tutorials — Here’s a list of tutorials for Pygame, a popular Python library for making games.
- Making Games with Pygame — A book that teaches you how to make games using Python.
- Invent Your Own Computer Games with Python — A book that walks you through how to make several games using Python.
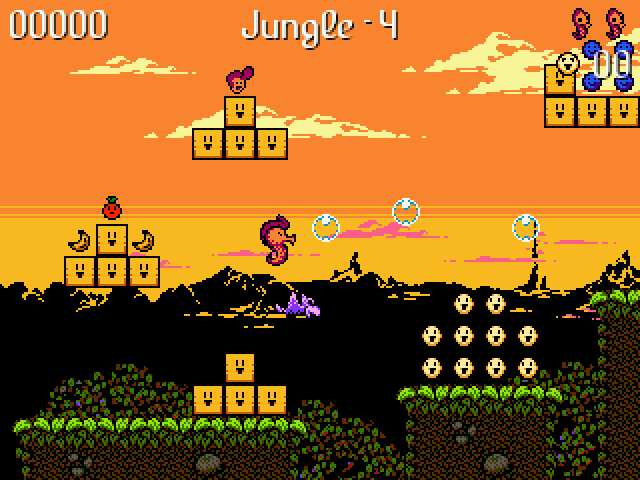
5. Hardware / Sensors / Robots
- Using Python with Arduino — Learn how to use Python to control sensors connected to an Arduino.
- Learning Python with Raspberry Pi — Build hardware projects using Python and a Raspberry Pi.
- Learning Robotics using Python — Learn how to build robots using Python.
- Raspberry Pi Cookbook — Learn how to build robots using the Raspberry Pi and Python.
6. Data Processing and Analysis
- Pandas Getting Started Guide — An excellent resource to learn the basics of pandas, one of the most popular Python libraries for data manipulation and analysis.
- NumPy Tutorials — Learn how to work with arrays and perform numerical operations efficiently with this core Python library for scientific computing.
- Guide to NumPy, pandas, and Data Visualization — A comprehensive collection of tutorials, practice problems, cheat sheets, and projects to build foundational skills in data analysis and visualization.
7. Automating Work Tasks
- Automate the Boring Stuff with Python — Learn how to automate day-to-day tasks using Python.
- Python Automation Cookbook — A book offering practical recipes for automating repetitive tasks, perfect for professionals looking to boost productivity with Python.
As I mentioned earlier, projects are where you do most of your actual learning. They stretch your capabilities, motivate you to learn new concepts, and allow you to showcase your abilities to potential employers. Once you’ve done a few structured projects, you can move on to working on your own projects.
Step 4: Work on Your Own Projects
After you’ve worked through a few structured projects, it’s time to kick things up a notch. Now, it's time to speed up learning by working on independent Python projects.
My advice: Start with a small project. It's better to finish a small project than embark on a huge one that is never finished.
Tips for Finding Independent Python Projects Ideas
I know it can feel daunting to find a good Python project to work on. Here are some tips for finding interesting ideas:
- Extend the projects you were working on before and add more functionality.
- Check out our list of Python projects for beginners.
- Go to Python meetups in your area and find people working on interesting projects.
- Find guides on contributing to open source or explore trending Python repositories for inspiration.
- See if any local nonprofits are looking for volunteer developers. You can explore opportunities on platforms like Catchafire or Volunteer HQ.
- Extend or adapt projects other people have made. Explore interesting repositories on Awesome Open Source.
- Browse through other people’s blog posts to find interesting project ideas. Start with Python posts on DEV Community.
- Think of tools that would make your everyday life easier. Then, build them.
Independent Python Project Ideas
1. Data Science and Machine Learning
- A map that visualizes election polling by state
- An algorithm that predicts the local weather
- A tool that predicts the stock market
- An algorithm that automatically summarizes news articles
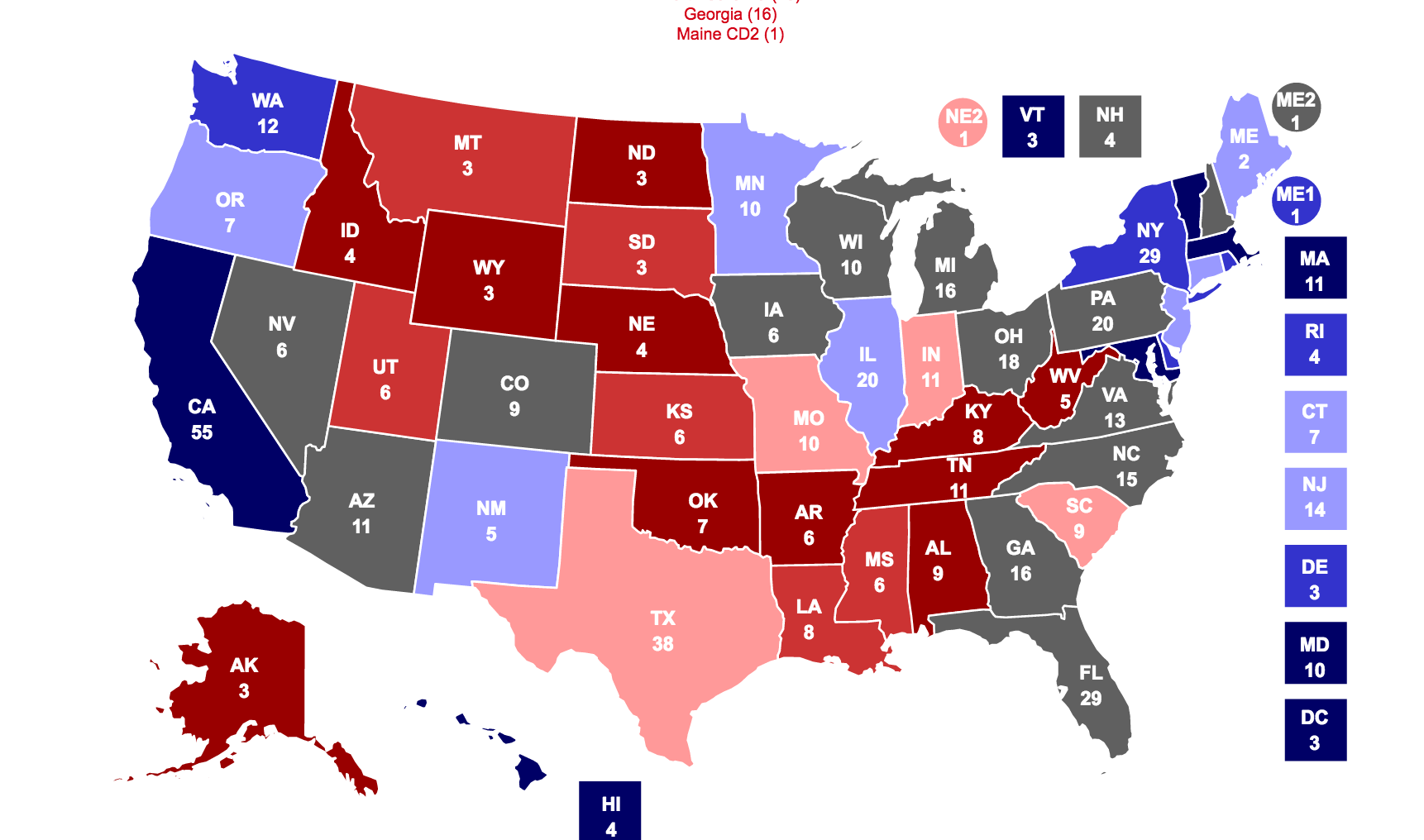
2. Mobile Apps
- An app to track how far you walk every day
- An app that sends you weather notifications
- A real-time, location-based chat
3. Website Projects
- A site that helps you plan your weekly meals
- A site that allows users to review video games
- A note-taking platform
4. Python Game Projects
- A location-based mobile game, in which you capture territory
- A game in which you solve puzzles through programming
5. Hardware / Sensors / Robots Projects
- Sensors that monitor your house remotely
- A smarter alarm clock
- A self-driving robot that detects obstacles
6. Data Processing and Analysis Projects
- A tool to clean and preprocess messy CSV files for analysis
- An analysis of movie trends, such as box office performance over decades
- An interactive visualization of wildlife migration patterns by region
7. Work Automation Projects
- A script to automate data entry
- A tool to scrape data from the web
The key is to pick something and do just it. If you get too hung up on finding the perfect project, you risk never starting one.
My first independent project consisted of adapting my automated essay-scoring algorithm from R to Python. It didn't look pretty, but it gave me a sense of accomplishment and started me on the road to building my skills.
Obstacles are inevitable. As you build your project, you will encounter problems and errors with your code. Here are some resources to help you.
Resources If You Get Stuck
Don’t let setbacks discourage you. Instead, check out these resources that can help:
- StackOverflow — A community question and answer site where people discuss programming issues. You can find Python-specific questions here.
- Google — The most commonly used tool of any experienced programmer. Very useful when trying to resolve errors. Here’s an example.
- Official Python Documentation — A good place to find reference material on Python.
- Use an AI-Powered Coding Assistant — AI coding assistants can save you a lot of time by avoiding having to search the web for your exact situation when you need a little extra help troubleshooting some problematic code.
Step 5: Keep Working on Harder Projects
As you find success with independent projects, keep increasing the difficulty and scope of your projects. Learning Python is a process, and you’ll need momentum to get through it.
Once you’re completely comfortable with what you’re building, it’s time to try something harder. Continue to find new projects that challenge your skills and push you to grow.
5 Ways to Know You Are a Pythonista
Here are some ideas for when that time comes:
- Try teaching a novice how to build one of your projects.
- Ask yourself: Can you scale your tool? Can it work with more data, or can it handle more traffic?
- Try making your program run faster.
- Imagine how you might make your tool useful for more people.
- Imagine how to commercialize what you’ve made.
Final Thoughts
Remember, Python is continually evolving. There are only a few people in the world who can claim to understand Python completely. And those are the people who created it!
Where does that leave you? In a constant state of learning and working on new projects to hone your skills.
Six months from now, you’ll look back on your code and realize how terrible it is. At that point, you’ll know you’re on the right track.
If you thrive with minimal structure, then you have all you need to start your journey. However, if you need a little more guidance, our courses may help.
I founded Dataquest to help people learn quickly and avoid the things that make people quit. Our courses will have you writing actual code within minutes and completing real projects within hours.
If you’re looking for the best way to learn Python from scratch and build a career as a business analyst, data analyst, data engineer, or data scientist, our career paths are designed to get you there. With structured lessons, hands-on projects, and a focus on real-world skills, you can go from complete beginner to job-ready in a matter of months.
Now it’s your turn to take the first step. See you in the code!