Path overview
In this path, you’ll explore the basics of Python programming from preparing data all the way to predicting trends from real-world data. You’ll learn the fundamentals of Python, how to use Jupyter notebooks, work with numerical and text data and basic object-oriented programming.
Best of all, you’ll learn by doing – you’ll write code and get feedback directly in the browser. You’ll apply your skills to several guided projects involving realistic business scenarios to build your portfolio and prepare for your next interview.
Key skills
- Programming with Python to perform complex statistical analysis of large datasets
- Using Jupyter Notebook to create and share documents
- Working with text, date, and time data
- Performing object-oriented and functional programming with Python
Path outline
Part 1: Python Basics for Data Analysis [4 courses]
Introduction to Python Programming 3h
Objectives- Write computer programs using Python
- Save values using variables
- Process numerical data and text data
- Create lists using Python
Basic Operators and Data Structures in Python 5h
Objectives- Use for loops to repeat processes and conduct data analysis
- Implement if, else, and elif statements in programming logic
- Employ logical and comparison operators in Python
- Develop and update Python dictionaries for data manipulation
- Construct frequency tables using dictionaries for data analytics
Python Functions and Jupyter Notebook 7h
Objectives- Write Python functions
- Debug functions
- Define function arguments
- Write functions that return multiple variables
- Employ Jupyter notebook
- Build a portfolio project
Intermediate Python for Data Science 8h
Objectives- Clean and analyze text data
- Define object-oriented programming in Python
- Process dates and times
The Dataquest guarantee
Dataquest has helped thousands of people start new careers in data. If you put in the work and follow our path, you’ll master data skills and grow your career.
We believe so strongly in our paths that we offer a full satisfaction guarantee. If you complete a career path on Dataquest and aren’t satisfied with your outcome, we’ll give you a refund.
Master skills faster with Dataquest
Go from zero to job-ready
Learn exactly what you need to achieve your goal. Don’t waste time on unrelated lessons.
Build your project portfolio
Build confidence with our in-depth projects, and show off your data skills.
Challenge yourself with exercises
Work with real data from day one with interactive lessons and hands-on exercises.
Showcase your path certification
Share the evidence of your hard work with your network and potential employers.
Projects in this path
Profitable App Profiles for the App Store and Google Play Markets
For this project, you’ll be a data analyst at a company that builds free, ad-supported Android and iOS apps. To drive revenue, you’ll analyze real app market data to find app profiles that attract the most users.
Exploring Hacker News Posts
For this project, we’ll be data analysts exploring Hacker News posts. We’ll use Python string manipulation, OOP, and date handling to analyze trends driving post popularity. Check out our Jupyter Notebook Guided Project if needed.
Learning resources
Grow your career with
Dataquest.
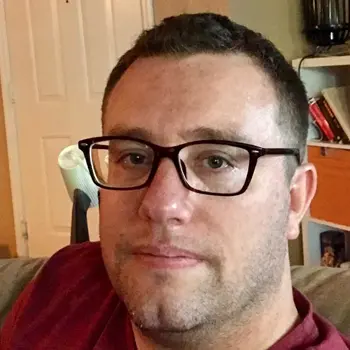
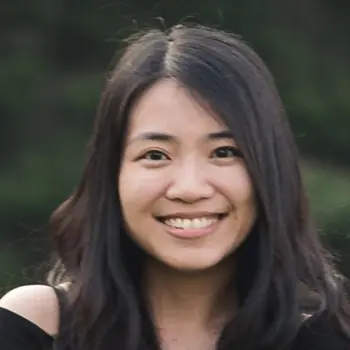
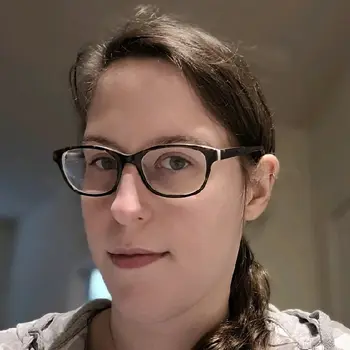