Course overview
In this course, you’ll learn about recursion, binary trees, binary heaps, and more. By the end, you’ll be able to explain the difference between iteration and recursion, build a binary heap to query large datasets, implement and query a dataset using Binary Search trees, and more.
Best of all, you’ll learn by doing – you’ll practice and get feedback directly in the browser. At the end of the course, you’ll complete a guided project in which you’ll use a B-Tree to implement a key-value datastore in Python.
Key skills
- Traversing tree data structures using recursion
- Using different types of tree data structures
- Explaining the various types of tree data structures
Course outline
Recursion and Trees for Data Engineering [8 lessons]
Overview of Recursion 2h
Lesson Objectives- Traverse tree data structures using recursion
- Identify the different types of tree data structures
- Implement different tree data structures
Introduction to Binary Trees 1h
Lesson Objectives- Define the binary tree structure
- Identify common algorithms used on the binary tree
Working with Binary Search Trees 1h
Lesson Objectives- Run a binary search tree
- Identify when to use a binary search tree over a heap
- Identify the runtime of binary search tree algorithms
Implementing a Binary Heap 1h
Lesson Objectives- Build a binary heap structure
- Determine which problems to solve using a binary heap
- Identify the runtime of binary heap algorithms
Performance Boosts of Using a B-Tree 1h
Lesson Objectives- Run a B-Tree
- Determine when to use a B-Tree over a binary search tree
- Implement important B-Tree algorithms
Implement a B-Tree data structure 1h
Lesson Objectives- Speed up search queries using an index
- Serialize Python objects to speed up algorithm loading
- Measure B-Tree algorithm speeds
Implementing a CSV Index with a B-Tree 1h
Lesson Objectives- Extend a class
- Build range queries for a B-tree
- Serialize python objects
Guided Project: Implementing a Key-Value Database 1h
Lesson Objectives- Adapt a data structure to create a database
- Implement a key-value database
Projects in this course
Implementing a Key-Value Database
For this project, we’ll step into the role of Python developers to build a key-value database using B-trees, enhancing it with features like range queries and dictionary-like syntax.
The Dataquest guarantee
Dataquest has helped thousands of people start new careers in data. If you put in the work and follow our path, you’ll master data skills and grow your career.
We believe so strongly in our paths that we offer a full satisfaction guarantee. If you complete a career path on Dataquest and aren’t satisfied with your outcome, we’ll give you a refund.
Master skills faster with Dataquest
Go from zero to job-ready
Learn exactly what you need to achieve your goal. Don’t waste time on unrelated lessons.
Build your project portfolio
Build confidence with our in-depth projects, and show off your data skills.
Challenge yourself with exercises
Work with real data from day one with interactive lessons and hands-on exercises.
Showcase your path certification
Share the evidence of your hard work with your network and potential employers.
Grow your career with
Dataquest.
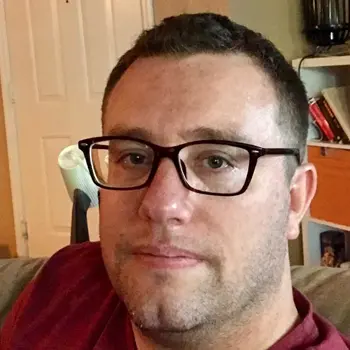
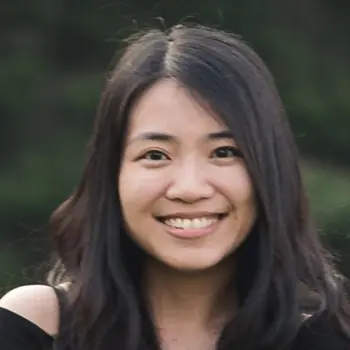
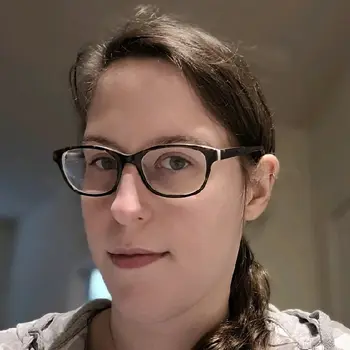