Course overview
In this course, you’ll learn several techniques for sampling data, such as random sampling and cluster sampling; you’ll also learn concepts such as discrete variables and random variables in the context of frequency distributions – and the different types of charts and graphs you might use to visualize frequency distributions.
Best of all, you’ll learn by doing – you’ll practice and get feedback directly in the browser. You’ll apply your skills to several guided projects involving realistic business scenarios to build your portfolio and prepare for your next interview.
In the guided project, you’ll investigate Fandango Movie Ratings to determine if Fandango is inflating movie ratings on its site. This project is a chance for you to apply the statistics skills you’ve learned and overcome common setbacks in practical data analysis.
Key skills
- Sampling data using simple, random, stratified and cluster sampling
- Measuring variables in statistics
- Building, visualizing, and comparing frequency distributions
Course outline
Introduction to Statistics in Python [6 lessons]
Variables in Statistics 2h
Lesson Objectives- Define variables in statistics
- Identify different kinds of variables
- Measure variables
Frequency Distributions 2h
Lesson Objectives- Define frequency distributions
- Generate frequency distribution tables
- Generate grouped frequency distribution tables
- Define proportions, percentages, and percentiles
Visualizing Frequency Distributions 1h
Lesson Objectives- Identify the importance of visualizing distributions
- Generate bar plots, pie charts, and histograms
- Employ bar plots, pie charts, and histograms
Comparing Frequency Distributions 2h
Lesson Objectives- Compare frequency distributions
- Define grouped bar plots
- Define step-type histograms
- Define kernel density estimate plots
- Define strip plots and box plots
Guided Project: Investigative Statistical Analysis - Analyzing Accuracy in Data Presentation 1h
Lesson Objectives- Expand your portfolio with a statistics project
- Identify common setbacks in practical data analysis
Projects in this course
Investigative Statistical Analysis - Analyzing Accuracy in Data Presentation
For this project, we’ll step into the role of data journalists to analyze movie ratings data and determine if there’s evidence of bias in Fandango’s rating system. We’ll apply statistical analysis skills using Python.
The Dataquest guarantee
Dataquest has helped thousands of people start new careers in data. If you put in the work and follow our path, you’ll master data skills and grow your career.
We believe so strongly in our paths that we offer a full satisfaction guarantee. If you complete a career path on Dataquest and aren’t satisfied with your outcome, we’ll give you a refund.
Master skills faster with Dataquest
Go from zero to job-ready
Learn exactly what you need to achieve your goal. Don’t waste time on unrelated lessons.
Build your project portfolio
Build confidence with our in-depth projects, and show off your data skills.
Challenge yourself with exercises
Work with real data from day one with interactive lessons and hands-on exercises.
Showcase your path certification
Share the evidence of your hard work with your network and potential employers.
Grow your career with
Dataquest.
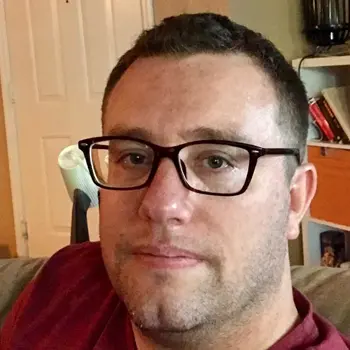
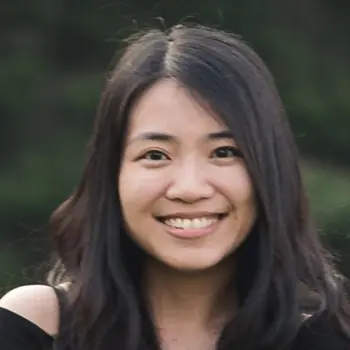
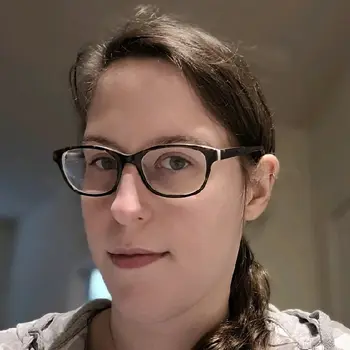