Course overview
This course is for intermediate Python users, and it builds upon the essentials covered in our previous Python lessons. You’ll learn how to leverage Python to supercharge your data analysis workflow. You’ll learn how to manipulate, combine, transform, and merge data; manipulate strings; and work with missing values in Python – as well as new concepts and techniques to improve the speed and efficiency of your Python code.
Best of all, you’ll learn by doing – you’ll practice and get feedback directly in the browser. You’ll apply your skills to several guided projects involving realistic business scenarios to build your portfolio and prepare for your next interview.
Key skills
- Aggregating, combining, transforming, and cleaning data
- Manipulating strings and working with regular expressions in Python
- Combining, transforming, and reshaping datasets
- Resolving missing data and duplicate data
Course outline
Data Cleaning and Analysis in Python [6 lessons]
Data Aggregation 2h
Lesson Objectives- Employ different techniques for aggregating data
- Generate intuition for the groupby operation
Combining Data Using Pandas 2h
Lesson Objectives- Employ different techniques for combining data
- Employ database-style joins and keys
Transforming Data with Pandas 2h
Lesson Objectives- Transform columns in pandas using custom functions
- Reshape data to prepare it for analysis
Working with Strings in Pandas 2h
Lesson Objectives- Manipulate strings with pandas
- Employ regular expressions
Working With Missing And Duplicate Data 2h
Lesson Objectives- Drop rows and columns with missing data
- Impute values to replace missing data
- Identify and drop duplicate rows
Guided Project: Clean and Analyze Employee Exit Surveys 3h
Lesson Objectives- Clean and analyze datasets
- Expand your portfolio with pandas
Projects in this course
Clean and Analyze Employee Exit Surveys
For this project, we’ll assume the role of data analysts for the Department of Education, Training and Employment and the Technical and Further Education institute in Queensland, Australia to analyze employee exit surveys and uncover insights about why employees resign.
The Dataquest guarantee
Dataquest has helped thousands of people start new careers in data. If you put in the work and follow our path, you’ll master data skills and grow your career.
We believe so strongly in our paths that we offer a full satisfaction guarantee. If you complete a career path on Dataquest and aren’t satisfied with your outcome, we’ll give you a refund.
Master skills faster with Dataquest
Go from zero to job-ready
Learn exactly what you need to achieve your goal. Don’t waste time on unrelated lessons.
Build your project portfolio
Build confidence with our in-depth projects, and show off your data skills.
Challenge yourself with exercises
Work with real data from day one with interactive lessons and hands-on exercises.
Showcase your path certification
Share the evidence of your hard work with your network and potential employers.
Grow your career with
Dataquest.
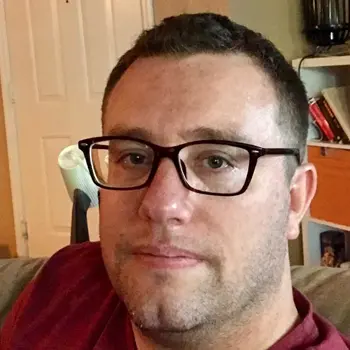
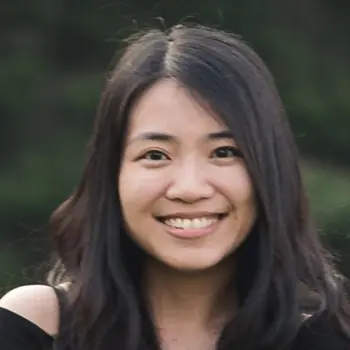
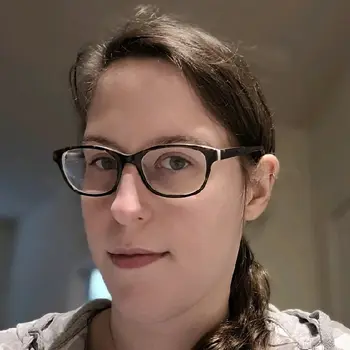