Course overview
Data cleaning is a necessary skill for anyone who wants to work in a data-related field.
You’ll start this course by learning how to identify data cleaning needs prior to analysis, how to use functionals for data cleaning, how to practice string manipulation, how to work with relational data, and how to reshape data using tools from the tidyverse. You’ll create correlation matrices to identify trends in your data, and then you’ll then learn how to deal with missing values in your dataset.
Best of all, you’ll learn by doing – you’ll practice and get feedback directly in the browser. At the end of the course, you’ll work on a guided project to analyze parents’, students’, and teachers’ perceptions of NYC schools. You’ll learn to work with survey data – specifically how to import, simplify, and reshape the data. You’ll also learn about R Notebooks and how you can use them to showcase your work.
Key skills
- Manipulating DataFrames with new tools
- Resolving missing data
- Joining DataFrames
- Reshaping data using the tidyr package
Course outline
Introduction to Data Cleaning in R [6 lessons]
Data Cleaning With R 2h
Lesson Objectives- Identify data cleaning needs prior to analysis
- Simplify DataFrames
- Change the data types of multiple variables at once
- Create new variables by calculating summary statistics from existing variables
- Employ functionals to check for duplicated observations
String Manipulation 1h
Lesson Objectives- Manipulate strings to create new variables
- Manipulate strings to Update variables
Relational Data 1h
Lesson Objectives- Define relational data, joins, and keys
- Combine DataFrames using joins
Correlations and Reshaping Data 1h
Lesson Objectives- Reshape data using tidyverse tools
- Identify relationships between variables using correlation analysis
- Identify trends in your data using correlation matrices
Dealing With Missing Data 1h
Lesson Objectives- Omit missing values from calculations
- Resolve missing values using different approaches
- Perform analysis when data is missing
Guided Project: NYC Schools Perceptions 1h
Lesson Objectives- Employ R Notebooks to showcase your work
- Reshape a large survey dataset
- Interpret metadata to inform your data cleaning
- Explore perceptions of NYC schools using data visualization and correlation
Projects in this course
NYC Schools Perceptions
For this project, you’ll become a data analyst using R Notebooks to clean, reshape and visualize NYC school survey data, uncovering insights into school quality perceptions.
The Dataquest guarantee
Dataquest has helped thousands of people start new careers in data. If you put in the work and follow our path, you’ll master data skills and grow your career.
We believe so strongly in our paths that we offer a full satisfaction guarantee. If you complete a career path on Dataquest and aren’t satisfied with your outcome, we’ll give you a refund.
Master skills faster with Dataquest
Go from zero to job-ready
Learn exactly what you need to achieve your goal. Don’t waste time on unrelated lessons.
Build your project portfolio
Build confidence with our in-depth projects, and show off your data skills.
Challenge yourself with exercises
Work with real data from day one with interactive lessons and hands-on exercises.
Showcase your path certification
Share the evidence of your hard work with your network and potential employers.
Grow your career with
Dataquest.
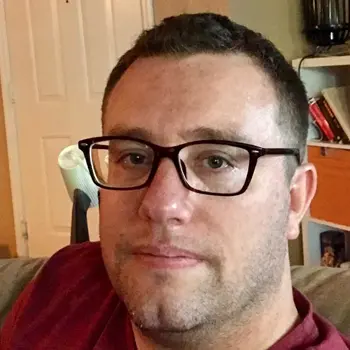
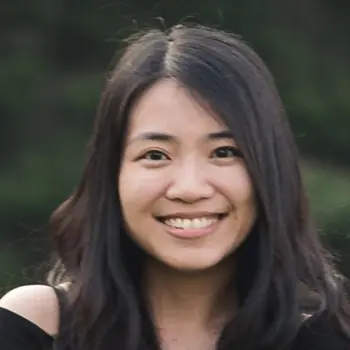
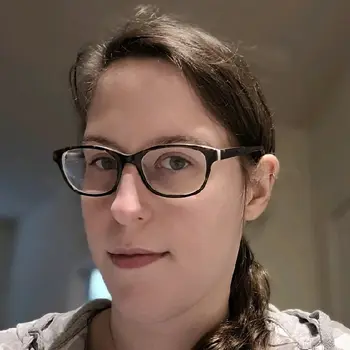