Course overview
In this course, you’ll learn how to develop a machine learning workflow for classification tasks using scikit-learn. You’ll learn how to build and implement the k-nearest neighbors algorithm using pandas and scikit-learn. Finally, you’ll learn to train, validate, and improve your machine learning model for better performance and accuracy using techniques like tuning hyperparameters.
Best of all, you’ll learn by doing — you’ll practice and get feedback directly in the browser. At the end of the course, you’ll combine your new skills to complete a project to predict heart disease.
Key skills
- Establishing a machine learning workflow
- Implementing the k-nearest neighbors algorithm for a classification task using pandas
- Implementing the k-nearest neighbors algorithm using scikit-learn
- Improving model performance using hyperparameters
Course outline
Introduction to Supervised Machine Learning in Python [5 lessons]
The Machine Learning Workflow 2h
Lesson Objectives- Define machine learning and supervised machine learning
- Establish a machine learning workflow
- Define classification and different types of classification tasks
- Build a linear SVM classifier (LinearSVC) using scikit-learn
- Compare the balance between applying an ML model with and without understanding the algorithm
Introduction to K-Nearest Neighbors 2h
Lesson Objectives- Explain how the K-Nearest Neighbors algorithm works for classification tasks
- Define the role of distance metrics for the K-Nearest Neighbors algorithm
- Implement the K-Nearest Neighbors algorithm for one feature using pandas
- Implement the K-Nearest Neighbors algorithm for multiple features using pandas
- Engineer new features to improve model performance
- Evaluate the model using accuracy as the metric
Evaluating Model Performance 2h
Lesson Objectives- Implement the K-Nearest Neighbors algorithm using scikit-learn
- Apply train/test validation
- Explain why validation is important for training models
- Interpret underfitting and overfitting
- Improve the model’s accuracy
Hyperparameter Optimization 2h
Lesson Objectives- Define a hyperparameter
- Identify how tuning hyperparameters impacts model performance
- Find optimal hyperparameter value by applying the grid search technique using scikit-learn
Guided Project: Predicting Heart Disease 2h
Lesson Objectives- Conduct exploratory data analysis on the dataset
- Clean and prepare the dataset for training a k-NN model
- Identify features of interest for training the model
- Build and train a classifier using selected features
- Find optimal hyperparameter values using grid search
- Evaluate the model's performance on the test set
Projects in this course
Guided Project: Predicting Heart Disease
Build a K Nearest Neighbors classifier to predict whether patients might be at risk of heart disease.
The Dataquest guarantee
Dataquest has helped thousands of people start new careers in data. If you put in the work and follow our path, you’ll master data skills and grow your career.
We believe so strongly in our paths that we offer a full satisfaction guarantee. If you complete a career path on Dataquest and aren’t satisfied with your outcome, we’ll give you a refund.
Master skills faster with Dataquest
Go from zero to job-ready
Learn exactly what you need to achieve your goal. Don’t waste time on unrelated lessons.
Build your project portfolio
Build confidence with our in-depth projects, and show off your data skills.
Challenge yourself with exercises
Work with real data from day one with interactive lessons and hands-on exercises.
Showcase your path certification
Impress employers by completing a capstone project and certifying it with an expert review.
Grow your career with
Dataquest.
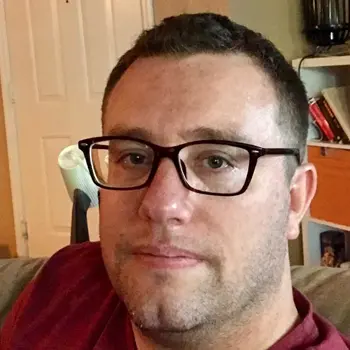
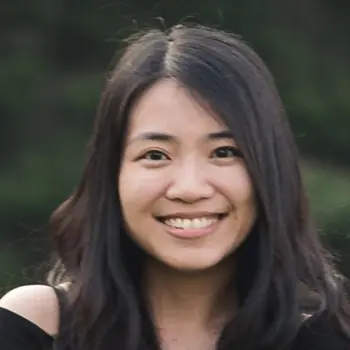
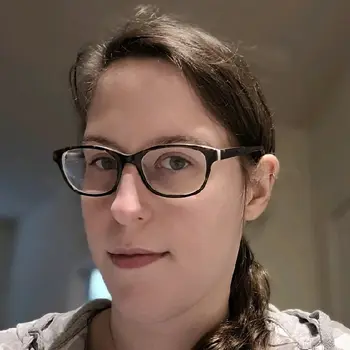