Course overview
Decision trees are known in the machine learning world for a particularly distinctive characteristic: their visualizations are easier to understand compared to other machine learning models, and for this reason, they are very suitable for explaining insights to non-technical audiences.
In this course, you’ll learn the foundations of Decision Trees including identifying the key components of trees, interpreting them, classifying new observations using decision trees and calculating optimal thresholds for both classification and regression trees. You’ll also learn how to build and visualize decision trees by adapting a real-life dataset to train tree models, selecting the appropriate scikit-learn tools to build your model, and training, testing and visualizing decision trees.
You’ll be able to evaluate and optimize trees for better performance including activities such as establishing the optimal depth for a decision tree, using Prune decision trees to avoid overfitting, or manipulating sample distribution in nodes and leaves.
Finally, you’ll learn how to apply the cross validation and ensemble techniques for decision trees. You’ll identify the differences between decision trees and random forest models, develop and customize random forest models and optimize the parameters of random forest.
Best of all, you’ll learn by doing – you’ll practice and get feedback directly in the browser. At the end of the course, you’ll combine your new skills in a project to predict employee productivity with tree models
Key skills
- Creating, customizing, and visualizing Decision Trees
- Using and interpreting Decision Trees on new data
- Optimizing trees by altering their parameters
- Applying the Random Forest prediction technique
Course outline
Decision Tree and Random Forest Modeling in Python [5 lessons]
Foundations of Decision Trees 2h
Lesson Objectives- Identify key components of trees
- Interpret decision trees
- Classify new observations using decision trees
- Calculate optimal thresholds for both classification and regression trees
Building Decision Trees Using Scikit-learn 2h
Lesson Objectives- Adapt a real-life dataset to train tree models
- Select the appropriate scikit-learn tools to build decision trees
- Train and test decision trees
- Visualize decision trees
Evaluating and Optimizing Decision Trees 2h
Lesson Objectives- Evaluate the trees' performance
- Optimize your trees
- Establish the optimal depth for a decision tree
- Prune decision trees to avoid overfitting
- Manipulate sample distribution in nodes and leaves
Cross Validation and Ensemble Techniques for Decision Trees 2h
Lesson Objectives- Apply cross validation techniques to decision trees.
- Find the optimal parameters of decision trees using grid search.
- Identify the differences between decision trees and random forest models.
- Develop and customize random forest models.
- Optimize the parameters of random forest.
- Identify the differences between random forest and extra trees.
- Determine the advantages and disadvantages of decision trees.
Guided Project: Predicting Employee Productivity Using Tree Models 1h
Lesson Objectives- Clean and adapt a dataset for use in a decision tree
- Build and visualize a decision tree to determine key features
- Evaluate your trees using different metrics
- Optimize trees by adjusting their parameters
- Explain the results of a tree model to a non-technical audience
Projects in this course
Predicting Employee Productivity Using Tree Models
For this project, we’ll step into the role of data scientists to determine the best working conditions for maximizing productivity in a garment factory using decision trees and random forests in Python.
The Dataquest guarantee
Dataquest has helped thousands of people start new careers in data. If you put in the work and follow our path, you’ll master data skills and grow your career.
We believe so strongly in our paths that we offer a full satisfaction guarantee. If you complete a career path on Dataquest and aren’t satisfied with your outcome, we’ll give you a refund.
Master skills faster with Dataquest
Go from zero to job-ready
Learn exactly what you need to achieve your goal. Don’t waste time on unrelated lessons.
Build your project portfolio
Build confidence with our in-depth projects, and show off your data skills.
Challenge yourself with exercises
Work with real data from day one with interactive lessons and hands-on exercises.
Showcase your path certification
Share the evidence of your hard work with your network and potential employers.
Grow your career with
Dataquest.
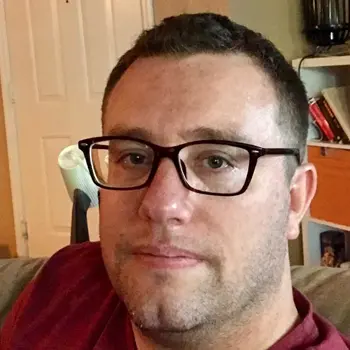
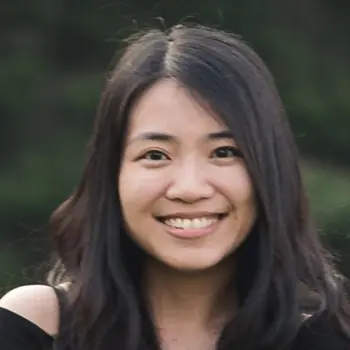
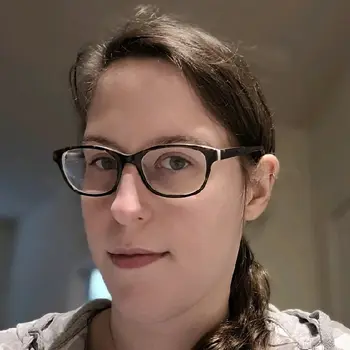