Course overview
This course builds on basic data cleaning knowledge and requires intermediate familiarity with Python for data science. You’ll learn how to clean and manipulate text data using basic and advanced regular expressions, how to resolve missing data, and how to employ lambda functions and list comprehension with pandas. Best of all, you’ll learn by doing – you’ll practice and get feedback directly in the browser.
Key skills
- Cleaning and manipulating text data using regular expressions
- Resolving missing data and duplicate data
- Employing lambda functions and list comprehension with pandas
- Advanced techniques for data cleaning
Course outline
Advanced Data Cleaning in Python [4 lessons]
Regular Expression Basics 2h
Lesson Objectives- Employ regular expressions
- Define regex components like character classes and quantifiers
- Employ regular expressions with the 're' module and pandas
Advanced Regular Expressions 3h
Lesson Objectives- Clean data by substituting regular expression matches
- Employ lookarounds to include and exclude text before and after your regex match
- Extract new columns from text data using capture groups
List Comprehensions and Lambda Functions 2h
Lesson Objectives- Read and employ JSON files
- Create and transform lists using List Comprehensions
- Create and employ lambda functions
Working with Missing Data 2h
Lesson Objectives- Identify missing data using both code and visualizations
- Resolve missing data using imputation
- Fill missing values using external data
The Dataquest guarantee
Dataquest has helped thousands of people start new careers in data. If you put in the work and follow our path, you’ll master data skills and grow your career.
We believe so strongly in our paths that we offer a full satisfaction guarantee. If you complete a career path on Dataquest and aren’t satisfied with your outcome, we’ll give you a refund.
Master skills faster with Dataquest
Go from zero to job-ready
Learn exactly what you need to achieve your goal. Don’t waste time on unrelated lessons.
Build your project portfolio
Build confidence with our in-depth projects, and show off your data skills.
Challenge yourself with exercises
Work with real data from day one with interactive lessons and hands-on exercises.
Showcase your path certification
Share the evidence of your hard work with your network and potential employers.
Grow your career with
Dataquest.
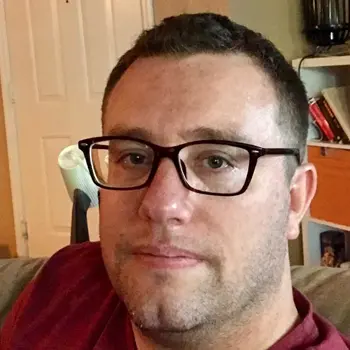
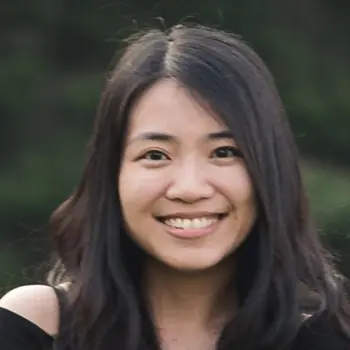
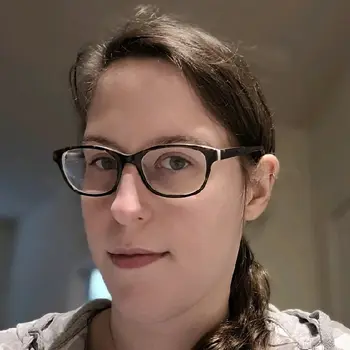